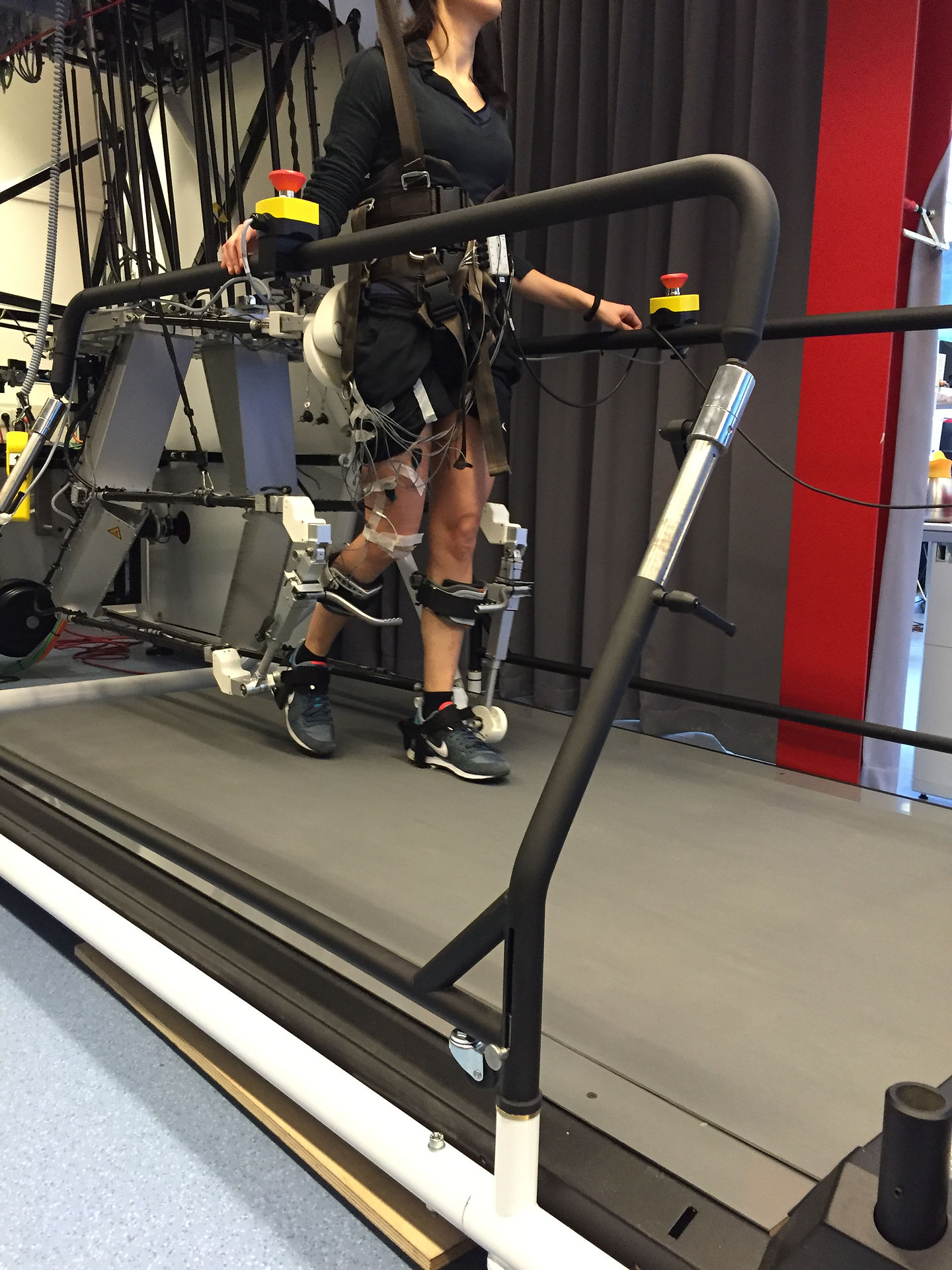
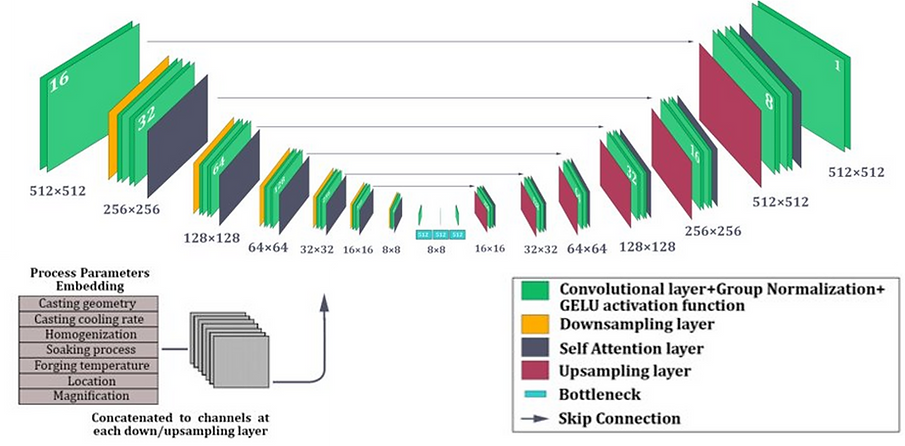
Predictive Modeling and Optimization in Material Science and Manufacturing
Generative Data-driven Models to Capture the Underlying Physics of Complex Manufacturing Processes, to Predict the Outcome Measures.
These Models are then Utilized in a Multi-objective Optimization Framework to Find the Pareto Front (set of optimal solutions) that a designer can choose from to guide the manufacturing process.

Generative AI for Synthetic High-fidelity Microscopic Images
We adapt the Denoising Diffusion Probabilistic Model structures to enable capturing the underlying physics of die-cast forging of Mg-alloys. The resultant tool can generate synthetic SEM images with high similarity to the real ones while capturing their microstructure features even for unseen conditions.
Denoising Diffusion Probabilistic Models (E. Azqadan, et al., Acta Materialia 2023)
To see how it works use this link to our dynamic Kaggle simulator
For implementation code, see the GitHub link
Data-driven Predictive Models for Manufacturing Performance Metrics
Data-driven models that can predict the outcome of complex Deform FEM analysis as well as hardness of Mg-alloy materials.
Data-driven prediction of forging outcome (T. Kodippili et al., Materials Today Comm 2022)
Mg-alloys Hardness variation in cast-forging process (E. Azqadan et al., Materials Today Comm 2023)
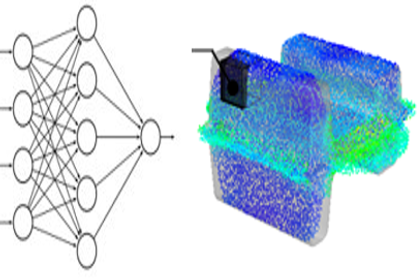
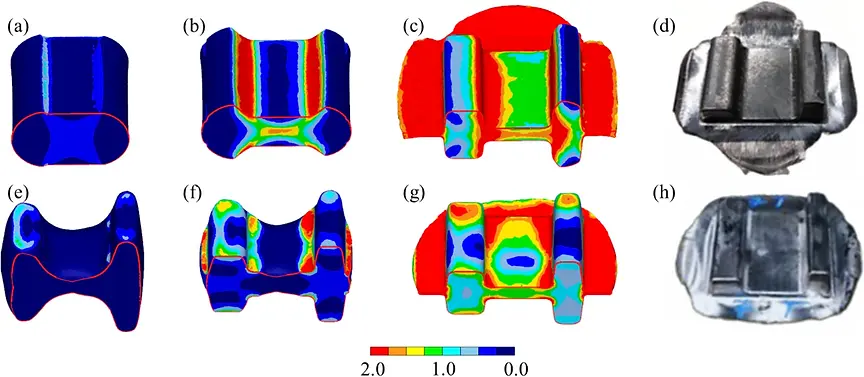
Multi-objective optimization of preform shape for hot forging
In a close collaboration with Fatigue and Stress Analysis Lab (led by Prof. H. Jahed) and Prof. S. Lambert and Prof. M. Wells, we are shaping the future of the manufacturing by using the power of AI and machine learning in prediction of manufacturing performance outcomes and optimizing them.
Multi-objective optimization of a cast-preform shape
(T. Kodippili, et al., Advanced Manufact. Tech. 2023)